Is your organization on top of all its data, or rather drowning in it? If you’re on a mission to become truly data-driven, you may need to consider switching to a modern data stack. The benefits it offers are nothing short of transformative, making it a critical asset for those companies who rely on data heavily and look for ways to boost efficiency, extend their decision-making capabilities, and overall competitiveness.
A modern data stack can empower an organization with the ability to harness data, both real-time and historical, to make informed choices that drive their strategies.
The benefits are multifold:
- improved business insights,
- competitive advantages,
- enhanced customer experiences,
- operational efficiency, scalability, and
- flexibility.
All these facets converge to deliver one overarching promise – staying ahead in the market race.
In this blog post, we present you with the modular data architecture that is the foundation for building a versatile data platform, capable of unlocking the aforementioned benefits regardless of your industry. Read on to discover why opting for a modern data platform by RST Data Cloud may be the game-changer you’re looking for.
What is a modern data stack?
The modern data stack refers to a set of technologies and tools used by organizations to collect, store, process, and analyze data with unprecedented efficiency and effectiveness. The data stack has evolved to address the challenges posed by the increasing volume, variety, and velocity of data in today's digital world.
Components of a modern data stack architecture
A modern data stack architecture typically includes the following components and capabilities:
Data sources
These are the various systems and platforms from which data is generated and collected. Sources can include databases (both SQL and NoSQL), web applications, IoT devices, APIs, logs, social media platforms, third party providers and more.
Data ingestion
Data from different sources needs to be collected and ingested into a central data repository. This can be done through simple bulk file uploads, streaming, ingestion from data lakes, databases as well as apps and SaaS tools and data sharing.

Data storage
Once ingested, data needs to be stored in a structured, semi-structured format or unstructured. Snowflake is a great option for this purpose as it allows storing different types of data.
Data transformation
Data transformation refers to refining the raw data into a format that can be used for analysis and reporting. It may involve data cleaning, formatting, derivation, etc. In the context of a modern data stack, data transformation involves the ELT process (Extract, Load, Transform), a data integration methodology that emphasizes that data is transformed after loading onto the data lakes or data warehouses.
This is a key distinction from ETL (Extract, Transform, Load) where data is transformed before loading. Opting for ELT allows for more effective processing or large volumes of data, and gives greater analytical flexibility and is cheaper, as it leverages the platform’s processing power.
Interactive computing
The modern data platform must allow two-way communication in order to accept user input. The interactive computing component is what assures the exchange between the user and the machine.
Data orchestration
This process is responsible for automating the management of the data flow within your organization. It essentially acts as a conductor, coordinating and streamlining the movement of data between various systems, applications, and data storage locations.
To automate data pipelines and workflows, tools like Apache Airflow, Luigi, or cloud-based solutions like AWS Step Functions or Google Cloud Composer are employed.
Data quality
As part of the modern data platform, the data quality component ensures the data we’re working with is always up to the desired standards. It encompasses various dimensions that ensure the data is reliable, trustworthy, and suitable for its intended purpose.
Cloud services
The choice of specific technologies and tools within the modern data stack can vary depending on an organization's size, data volume, budget, and specific use cases. However, the key goal is to enable data-driven decision-making and insights by providing a robust and flexible infrastructure for managing and analyzing data with the above capabilities.
Data stack vs data platform
The terms “data stack” and “data platform” are sometimes used interchangeably, but they can have different meanings depending on context. Here are the key differences between a data stack and a data platform:
Data stack
A data stack typically refers to the collection of technologies and tools used for specific data-related tasks within an organization, such as data ingestion, storage, transformation, analysis, and visualization. It is more technically oriented and often encompasses a set of individual components and services.
A data stack is often modular, consisting of various elements that serve specific functions. For example, it may include parts like data warehouses, data lakes, ETL pipelines, analytics tools, and visualization platforms.
A data stack is primarily focused on the selection and integration of tools and technologies that enable data-related tasks. It may not necessarily provide a unified or cohesive user interface or experience but rather a collection of tools that work together.
Organizations have the flexibility to customize their data stack by selecting the most suitable tools and components based on their use cases, data sources, and technology preferences.
Data platform
A data platform is a more comprehensive and integrated solution designed to address a broader range of data-related needs within an organization. It encompasses various tools and technologies but focuses on providing a unified environment for data management and utilization. As such, a data platform is when a data stack is put to its predefined use.
A data platform typically covers the entire data lifecycle, from data ingestion and storage to transformation, analysis, and reporting. It often includes components for data governance, security, collaboration, and metadata management.
A data platform aims to provide a consistent and user-friendly experience for data professionals and business users. It may offer a central interface or portal where users can access and interact with data, perform analytics, and collaborate.
Data platforms are designed to scale with growing data volumes and evolving business needs. They often support integration with various data sources, analytics tools, and external systems, offering a more cohesive and extensible solution.
Modern data stack vs traditional data stack
With the unprecedented pace of technology evolution, we shouldn’t be surprised to see the traditional data stack evolve into a more modern version. In fact, this development brings even more advantages for data-driven organizations than the traditional data platform could offer. The modern data stack is better positioned to cater to the fast-paced and diverse data needs of contemporary organizations.
Consider the below:
- Scalability: modern data stacks leverage cloud-based infrastructure and scalable technologies, allowing for efficient handling of large data volumes. Traditional stacks, often reliant on on-premises infrastructure, struggle with scalability.
- Data variety: while traditional data stack focuses primarily on structured data, modern data stacks accommodate diverse data types, including unstructured and semi-structured data, making them more versatile.
- Real-time processing: modern data stack embraces real-time and streaming data processing, enabling timely insights and enhancing data-driven applications. Traditional stacks often rely on batch processing, limiting real-time capabilities.
- Cost and maintenance: modern data stack offers a cost-effective approach by utilizing cloud-based solutions with pay-as-you-go pricing, eliminating heavy upfront investments and simplifying infrastructure management.
- Flexibility and agility: modern stack prioritizes flexibility, allowing easy integration of new data sources and technologies. They employ modular architectures, enabling rapid adaptation to evolving requirements. Traditional stacks can be rigid and less adaptable.
- Data democratization: modern data stack promotes data democratization, making data accessible to non-technical users through user-friendly tools, fostering a data-driven culture, and enabling more informed decision-making.
If your current data stack struggles to keep pace with the above requirements, it may suggest it’s just about time to modernize it.
The ultimate data stack for a versatile data platform

Data ingestion: Fivetran
Fivetran is a cloud-based data integration platform that automates the process of extracting data from various sources, transforming it from one format to another, and subsequently loading it into a destination data storage platform. In fact, it can load the data onto different locations. It plays a crucial role in our modern data stack by streamlining data integration and allowing our customers to access and analyze the data quickly and efficiently.
Role in RST Data Cloud
Fivetran takes the lead when it comes to ingesting the data into our modern data platform. We use it for two processes:
- Data integration: Fivetran automates the process of data integration and connects to a full spectrum of data sources, including databases, applications, SaaS platforms, and cloud services. It supports a wide range of source systems, such as Salesforce, Google Analytics, MySQL, PostgreSQL, and more, so will be instrumental in facilitating a smooth transition to RST Data Platform.
- Automation of data pipelines: Fivetran not only helps with data ingestion, but also builds and manages data pipelines that extract data from source systems. We use it for any necessary transformations and loading it into Snowflake, the data warehousing component. This automation reduces the time and effort required to set up and maintain data pipelines.
- Batch data updates: for data synchronization in batches
Why Fivetran?
It’s a managed SaaS, which takes away all the maintenance worries. Plus, Fivetran is the leading data ingestion tool currently available on the market.
Alternatives
While Fivetran is a popular choice for data ingestion, there is one alternative that we find particularly useful in our modern data stack, namely, Airbyte. It’s also designed to connect, collect, and synchronize data from various sources and make it available for analysis and decision-making. It simplifies the ELT processes from a wide range of data sources.
Airbyte is an open-source tool that does offer a SaaS component, but typically has to be installed manually. If used strategically with selected workloads, it can contribute to minimizing the operational cost of the modern data platform. It’s also a great choice for clients who want to limit the number of cloud services in their data stacks.
Data ingestion: Apache Kafka
A modern data platform must offer real-time data analytics capabilities. Kafka, an open-source distributed streaming platform, designed specifically for real-time data streaming and event processing is thus an indispensable component in our platform. It’s capable of dealing with high volumes of data and enabling real-time data processing and analysis.
Role in the data platform
Similarly to Fivetran, the RST Data Platform relies on Kafka for two processes:
- Data ingestion: Kafka serves as a central data ingestion layer, where data from various sources, such as applications, sensors, databases, and logs, can be ingested in real-time. It simply ensures that event data is efficiently collected and made available for processing.
- Real-time event streaming: Kafka is ideal for streaming events and messages in real-time. It enables the transmission of data between producers (data sources) and consumers (applications, databases, analytics platforms) with low latency and high throughput, which are crucial for efficiency in modern data platforms.
Why Kafka?
It’s the only reliable tool currently available for processing event data in real-time. The truth is: Kafka could take care of the entire data ingestion process, but using Fivetran and/or Airbyte is an effective and reliable way to cut costs. Relying solely on Kafka would greatly increase the data platform usage costs. Giants such as Netflix or LinkedIn have Kafka in their data stacks.
Alternatives
There aren’t any!
Data cloud: Snowflake
Snowflake, an innovative cloud-native data warehousing platform, plays an indispensable role in a modern data stack. Designed to manage and analyze large volumes of data in a completely novel way. It’s a lot more than a typical data warehouse: it serves as a central repository for data that further supports its processing, analysis and reporting. Role in the data stack
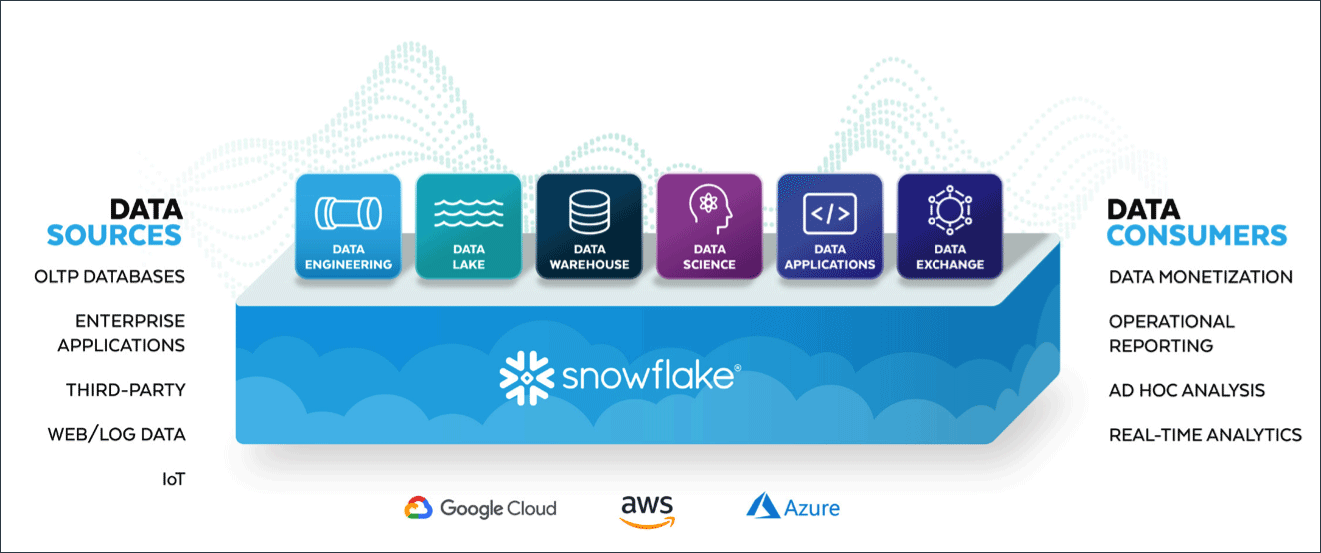
Snowflake is an all-in-one platform when it comes to managing and analyzing data efficiently. We use it exactly for what it has been designed for:
- Data engineering: Snowflake offers powerful data engineering capabilities for ingestion, transformation, and preparation for analysis. Additionally, Snowflake supports SQL-based transformations and data manipulation tasks, allowing data engineers to clean, enrich, and structure data according to business requirements.
- Data lake: With Snowflake's architecture, users can seamlessly store structures, semi-structured and unstructured data in a centralized repository. Snowflake's support for semi-structured data formats like JSON, Avro, and Parquet empowers users to leverage the benefits of a data lake alongside traditional data warehousing features.
- Data warehousing: Snowflake’s highly scalable and fully managed data warehousing solution in the cloud allows users to store data from various sources in a centralized location. One of its greatest advantages is that it allows businesses to access it for analytics and reporting faster, cheaper and more conveniently than other data warehousing platforms.
- Data science: Snowflake integrates seamlessly with popular data science tools, enabling data scientists to analyze data directly within the Snowflake platform. Snowflake's support for machine learning libraries and frameworks further enhances its capabilities for data science tasks, allowing users to build and deploy advanced analytics models at scale.
- Data application: Snowflake provides capabilities for building data-driven apps. Thanks to its robust SQL support and scalable performance, developers can build real-time analytics applications, interactive dashboards, and data-driven workflows directly on top of the Snowflake platform. Snowflake's cloud-native architecture and seamless integration with popular development frameworks simplify the process of building and deploying data applications in the cloud.
- Data exchange and collaboration: Snowflake enables organizations to securely share and exchange data with external partners, customers, and vendors. Its Data Exchange feature allows users to discover and access third-party data sets directly within the Snowflake platform. This capability streamlines data sharing and collaboration, as well as access to external data sources for enriched analytics.
Why Snowflake?
We love Snowflake because of its simplicity. We could use AWS or Azure with their respective components (Redshift and Synapse Analytics), but, since these are part of more robust and complex architectures with multiple dependencies, they are more demanding in terms of management. Knowledge of Redshift and Synapse requires a more extensive knowledge and skills when it comes to managing their respective cloud environments.
In contrast, Snowflake can easily be managed by analysts well versed with SQLs only via its robust yet simple documentation. This is a significant advantage from the end user’s perspective. Above all, Snowflakes focuses specifically on data warehousing, and that greatly simplifies its usage in this capacity.
In addition, Snowflake is cloud-agnostic, which greatly simplifies analytical processes for those clients who have their data sources dispersed across different cloud providers.
Alternatives
Snowflake is a popular choice for data warehousing and analytics. While Google BigQuery, Amazon Redshift or Microsoft Azure Synapse Analytics (formerly SQL Data Warehouse) could be used in its place to match business preferences, Snowflake would always be a superior choice when it comes to building modern data platforms.
Data transformation: dbt (data build tool)
dbt is an open-source command-line tool that provides a modeling layer in modern data platforms. dbt is designed to help data analysts and engineers transform raw data into structured, analysis-ready data sets.
Role in the data platform
In the context of data transformation and analytics, we use dbt for the following purposes:
- Automating data pipelines: dbt allows users to modularize their data transformations into reusable SQL-based models called “dbt models.” These models encapsulate specific business logic and transformations, making it easy to build and maintain complex data pipelines. dbt also automatically manages dependencies between different dbt models, supports incremental data builds and facilitates collaboration among team members.
- Data transformation: dbt focuses on data transformation and modeling. It allows data professionals to define, document, and test data transformation logic in SQL files. This SQL code is used to transform raw data into a structured and analytically useful format.
- Testing: dbt provides built-in testing capabilities that allow writing automated tests to validate data quality and transformations.
- Data lineage: dbt automatically captures and maintains data lineage information as part of the data transformation process. This lineage information tracks the flow of data from source to destination through various dbt models, providing visibility into how data is transformed and derived within the data pipeline.
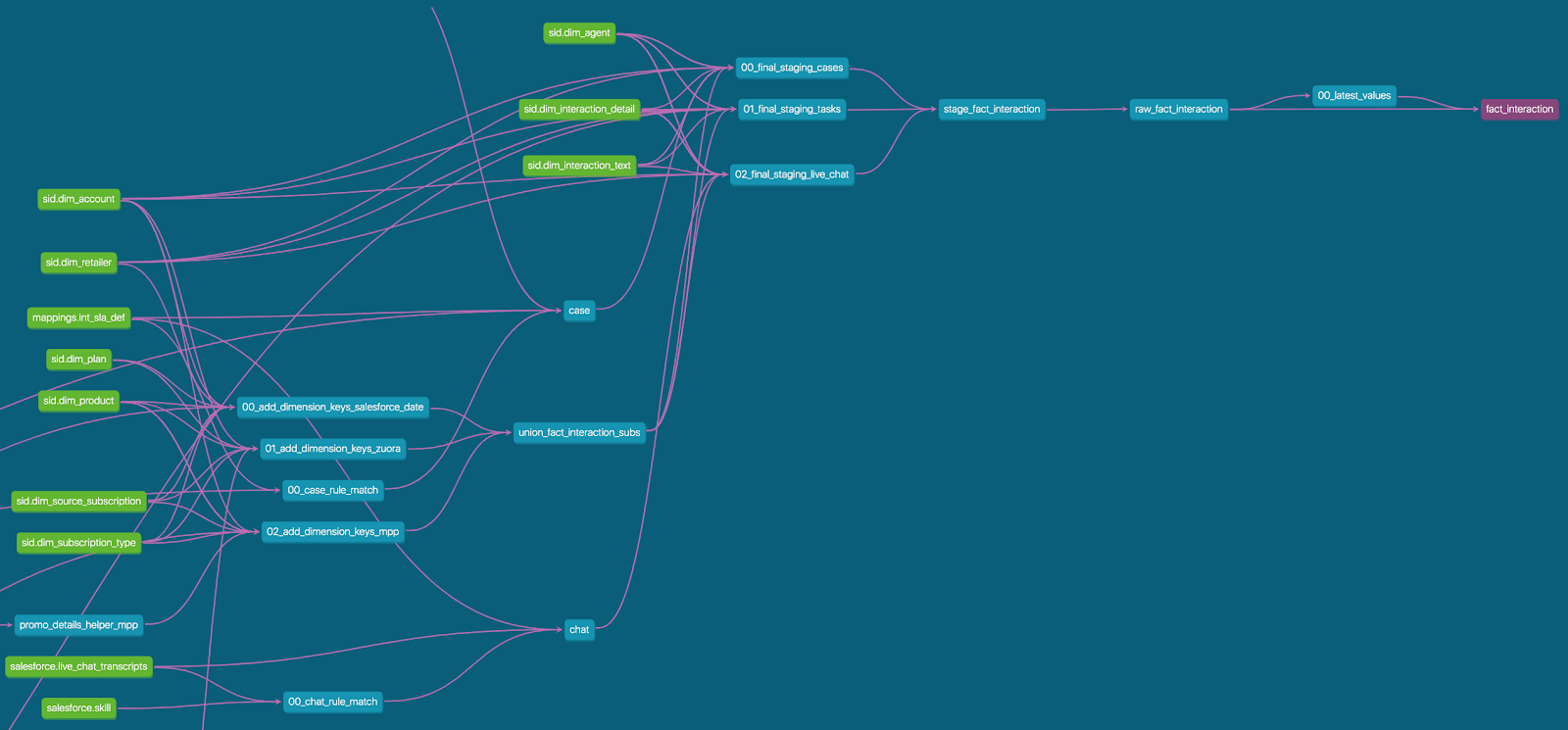
Why dbt?
In addition to data transformation and modeling, dbt is capable of data quality assurance (which we describe further below), so it allows us to have our cake and eat it.
Alternatives
We haven’t found any tool that would work better than dbt in the context of building a modern data platform from scratch.
Interactive computing: jupyter
Another open-source web application in our stack, jupyter allows to create and share documents containing live code, equations, visualizations, and narrative text. Jupyter plays a crucial role in a modern data stack by providing an interactive environment for data exploration, analysis, and collaboration.
Role in the data platform
Used primarily to enable ML and data science capabilities, we use jupyter for the following:
- Data exploration and analysis: Jupyter notebooks provide an interactive environment for data scientists, analysts, and engineers to explore data, run queries, and perform statistical analysis. They can execute code cells interactively, visualize data, and iterate on data analysis workflows.
- Collaborative work: Jupyter notebooks support collaboration by allowing multiple users to work on the same document simultaneously. Users can share notebooks via email, GitHub, or other platforms, enabling collaboration and knowledge sharing among team members.
Why jupyter?
It can visualize data in the data science capacity.
Alternatives
We haven’t found any.
Business intelligence: Tableau
Tableau is a widely popular data visualization and BI tool that is used to present the insights derived from data analysis. It allows users to connect to various data sources, create interactive and shareable dashboards, and subsequently demonstrate insights from data through visualizations.
Role in the data platform
We use Tableau in presenting the insights of the data processing and analyses that take place within specific data pipelines, through the following:
- Data visualization and reporting: this is what Tableau was created for. It allows end users to create interactive charts, graphs, maps, and dashboards to present data in a visually appealing and easy-to-understand format. It’s an invaluable tool for facilitating the communication of data insights effectively to stakeholders and decision-makers.
- Real-time analytics: Tableau supports real-time data connections, allowing users to create dashboards and visualizations that update in real-time as new data becomes available. This is valuable for monitoring and decision-making in dynamic environments.
- Advanced analytics: Tableau supports advanced analytics and predictive modeling through integrations with statistical tools and machine learning platforms. Users can incorporate advanced analytics results into their dashboards.
Why Tableau?
It’s one of the leading solutions on the market. Its dashboards and visualization options are highly customizable, visually appealing and simple to use.
Alternatives
Alternatives to Tableau in a modern data stack can include:
Cloud infrastructure: AWS
AWS doesn’t require an introduction. Naturally, a modern data platform is rooted in the cloud. We use it because, as a certified AWS partner, we know its services and capabilities inside out. We would like to draw your attention to four AWS tools that play a vital role in modern data stacks.
Role in the data platform
As the “host” of our modern data stack, AWS plays a number of critical roles:
- Containerized data workloads: we use AWS EKS to run containerized data workloads. Containerizing data processing tasks, ELT jobs, or data analytics applications and deploying them on EKS clusters adds scalability and flexibility.
- Data storage: AWS S3 can be used alongside Snowflake for storing data. It can store large volumes of structured, semi-structured, and unstructured data, including files, documents, images, videos, and more. Data can be organized into buckets and folders for efficient management.
- Data lake: S3 is a core component of data lake architectures. It allows building centralized repositories for raw and curated data for subsequent processing.
- Machine Learning models development: here, SageMaker provides a comprehensive set of tools and resources for data scientists and developers to build and train machine learning models. It supports a wide range of machine learning frameworks and algorithms, making it suitable for diverse use cases.
- Data preparation and preprocessing: SageMaker also offers data preprocessing capabilities, including feature engineering and data transformation. Data can be prepared within SageMaker for training machine learning models.
- Data processing: AWS Lambda can be used to process and transform data as it arrives in a data store, such as an Amazon S3 bucket or a streaming service like Amazon Kinesis. For example, Lambda functions can clean, enrich, or validate data in real-time.
- Data streaming: combining services like Snowpipe with Simple Notification Service and Simple Queue Service can be used to create a data pipeline for near-real-time data processing.
- ETL (Extract, Transform, Load): Lambda functions can serve as the "T" (Transform) in ETL processes. They can extract data from various sources, transform it according to business logic, and load it into a target data warehouse or data lake.
Cloud infrastructure: Terraform
Terraform is an open-source infrastructure as code (IaC) tool developed by HashiCorp. It is used to provision and manage infrastructure resources as code, enabling automation, version control, and reproducibility of infrastructure deployments.
Role in the data platform
Terraform plays a pivotal role in our data stack by automating the provisioning and configuration of infrastructure components.
- Infrastructure provisioning: Terraform allows you to define and provision infrastructure resources such as virtual machines, databases, networking components, and cloud services. This is particularly useful when setting up the foundation of a modern data stack in the cloud.
- Snowflake management: Terraform can be used to provision Snowflake databases, warehouses, schemas, tables, views, and other resources. Terraform allows you to configure various Snowflake parameters and enables you to manage access control by defining roles, users, and privileges in Terraform configuration files.
Why Terraform?
Terraform allows operators to define the entire data platform as infrastructure-as-code. This makes it consistent, versioned and reusable across environments.
Alternatives
While Terraform is a widely adopted IaC tool, there are alternative tools for automating infrastructure provisioning:
Data orchestration: Apache Airflow
Apache Airflow is an open-source platform used for orchestrating complex workflows and data pipelines. It allows organizations to schedule, monitor, and manage a wide range of tasks, making it a crucial component in modern data stacks.
Role in the data platform
Comprehensive management of entire data pipelines, which is achieved through the following:
- ELT pipeline orchestration: Airflow is commonly used to orchestrate ELT processes, ensuring that data is extracted from source systems, loaded into data warehouses or data lakes on a predefined schedule and transformed according to business logic.
- Data workflow automation: It automates and schedules various data-related tasks, such as data ingestion, data cleansing, data transformation, model training, and reporting, ensuring timely and consistent execution.
Why Airflow?
While Airbyte and dbt can of course be used to automate certain components of processes within the pipeline, applying Airflow for this purpose allows us to comprehensively manage entire data pipelines.
Alternatives
Alternatives to Apache Airflow in a modern data stack can include:
Data quality: dbt
Ensuring the appropriate quality of data is yet another crucial component of a modern data platform. We have already talked about dbt earlier when discussing data transformation. We also use it for its quality assurance capacity.
Role in the data platform
- Testing: dbt provides built-in testing capabilities that allow writing automated tests to validate data quality and transformations.
Alternative:
Modernize your cloud data platform with RST Data Cloud
In a landscape where data is the new currency, equipping yourself with the knowledge to build and optimize your data stack is not just an option – it's a necessity. We invite you to leverage this guide as a blueprint for your journey towards digital transformation. Our hope is that we’ve shed some light on how to harness the power of your data so that instead of drowning in it, you become empowered to make informed decisions that drive growth, efficiency, and competitive advantage.
Should you have any questions or wish to delve deeper into how these insights can be tailored to your unique organizational needs, our team at RST Data Cloud is here to illuminate the path forward. We specialize in crafting custom data solutions that help businesses achieve their strategic goals, ensuring that your journey through the realm of data is not just successful, but transformative.
If you require support with building or automation of any of the modern data platform components or processes, we are happy to share our expertise as required – we can adapt to your needs.
Contact us directly via this form to book a free consultation.