Thank you! Your submission has been received!
Oops! Something went wrong while submitting the form.
Access and follow quality content
by our team of writers.
Most recent
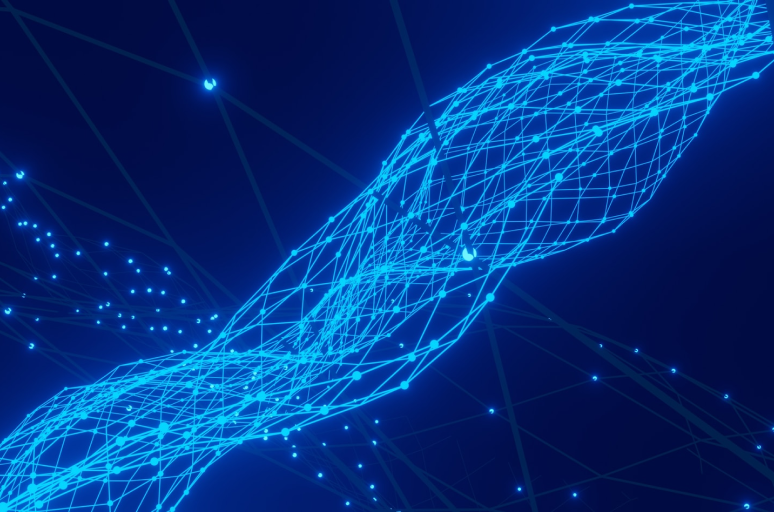
Data
Migrating data to Snowflake – best practices
Master Snowflake migration with RST Software’s best practices guide. Ensure a smooth transition and optimize your data platform’s performance.
Editor's choice
Latest articles
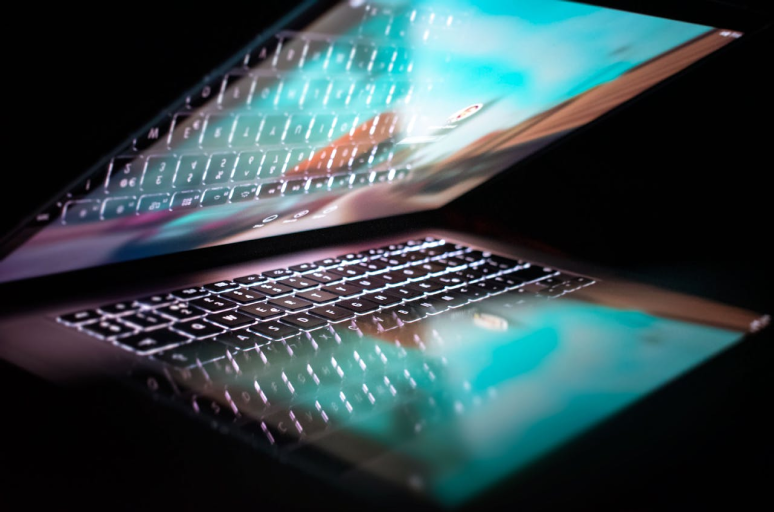
Data
Data access control in Snowflake: key principles and best practices
Secure your data with RST Software’s guide on Snowflake data access control. Learn to implement robust permissions and security measures.
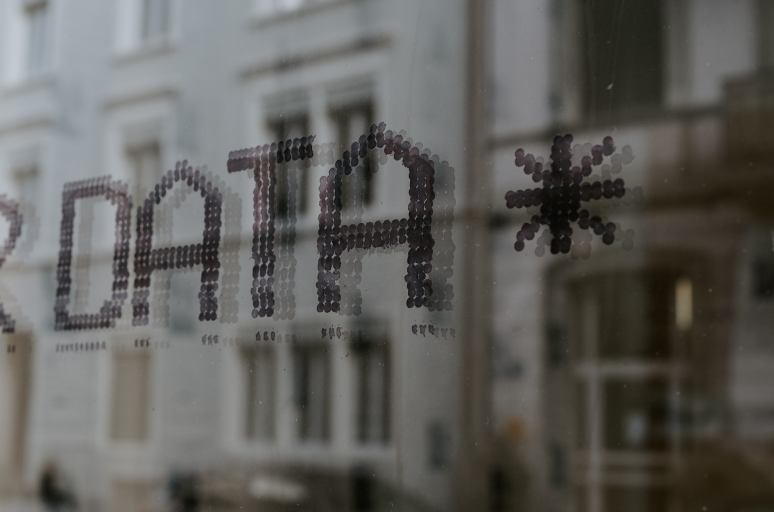
Data
The ultimate data glossary: 103 must-know terms
Navigate the world of data with our comprehensive data glossary. Understand key terms and concepts to enhance your expertise.
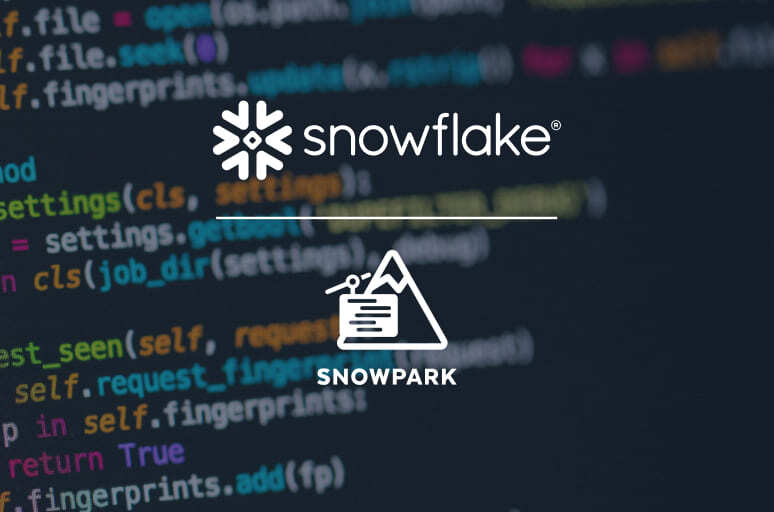
Data
What is Snowflake Snowpark and what does it bring to a modern data platform?
Explore Snowflake Snowpark. Discover its features and how it enhances modern data platforms for advanced analytics.
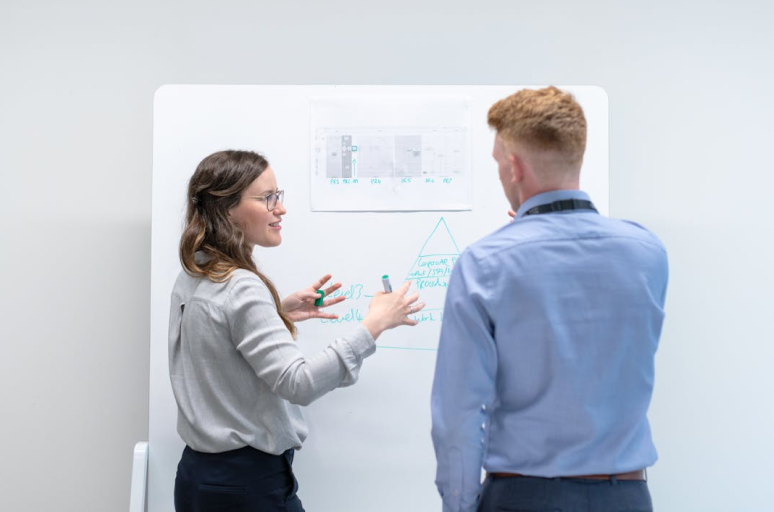
Data
How to build an actionable data strategy?
Learn to build a comprehensive data strategy. Gain insights on structuring your data for optimal business impact.
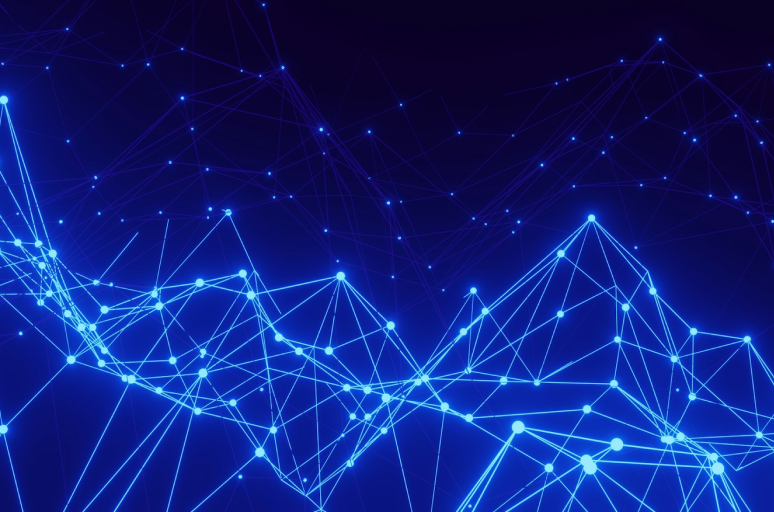
Data
Data science explained
Explore data science with RST Software's guide. Discover key concepts, tools, and applications to enhance your projects. Perfect for enthusiasts and professionals.
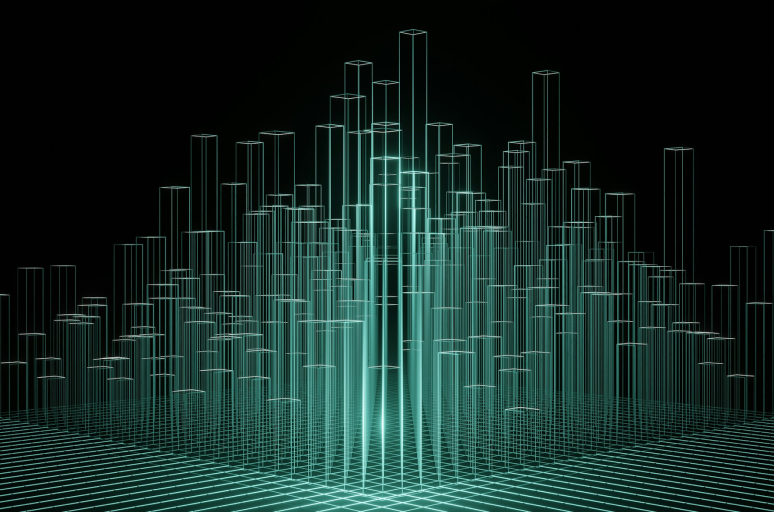
Data
The role of AI in data science in 2024
Dive into the future of AI in data science for 2024. Uncover trends, innovations, and how they're transforming industries.
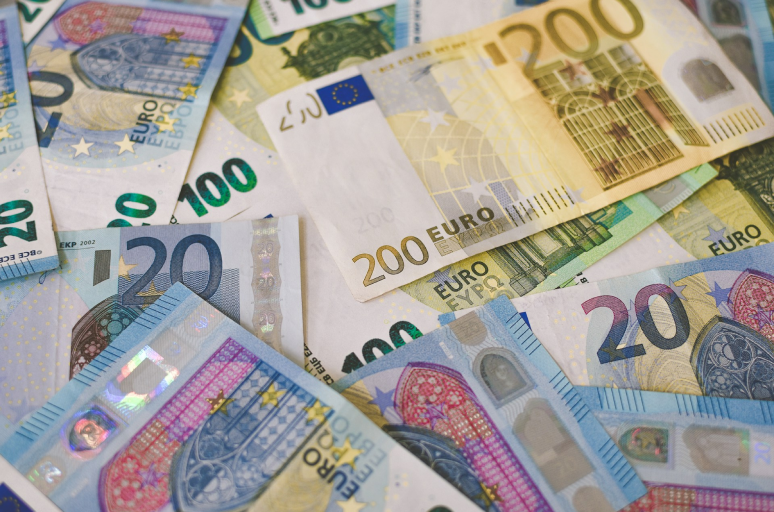
Data
Data monetization: turn your data into revenue streams
Unlock the secrets of data monetization. Learn how to transform your data into profitable revenue streams effectively.
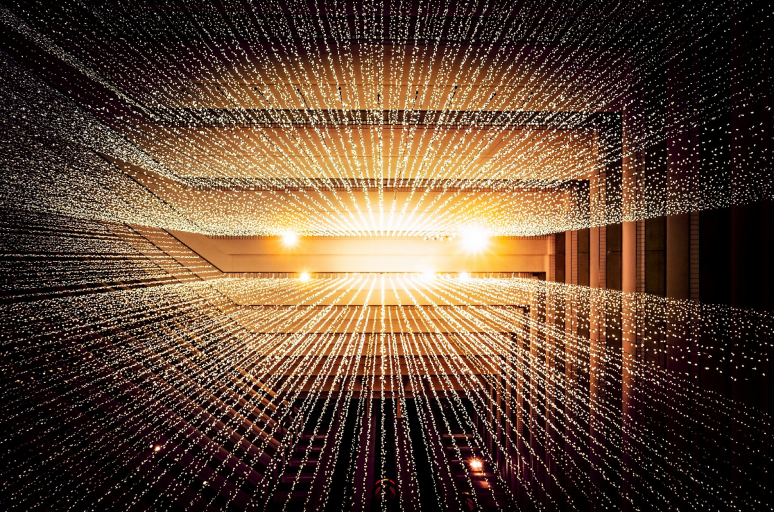
Data
Data governance strategy: building a framework for data-driven success
Master data governance with our strategy guide. Build a robust framework for data-driven success in your organization.
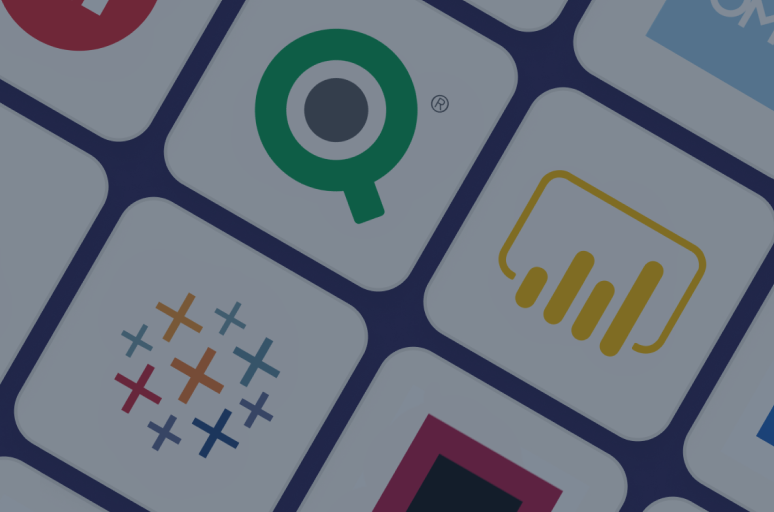
CEO Corner
Top Business Intelligence tools - free 2024 comparison
Explore the best free business intelligence tools for 2024. Compare features and functionalities to make informed choices.
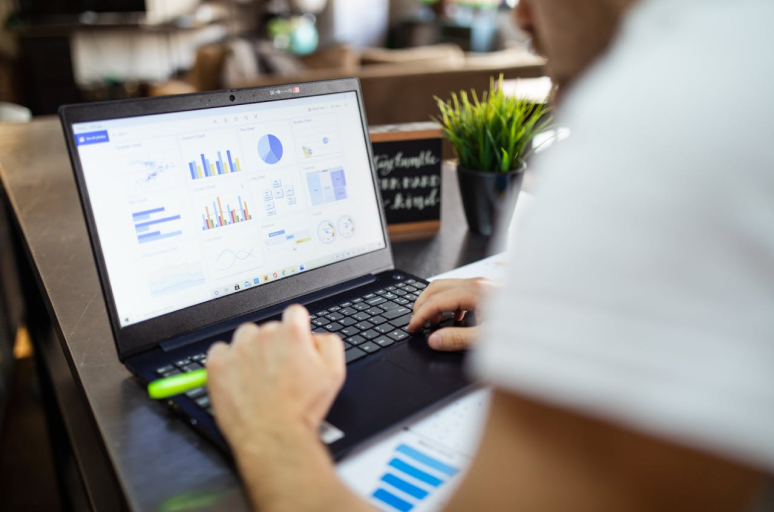
CEO Corner
How to pick a data analytics consultancy? Practical tips
Unlock practical tips for selecting the right data analytics consultancy. Make informed decisions to drive data-driven success.
.png)
CEO Corner
The data stack you’ll need to build a versatile modern data platform in 2024
Discover the essential components of the modern data stack for building a robust data platform in 2024. Stay ahead in data management.
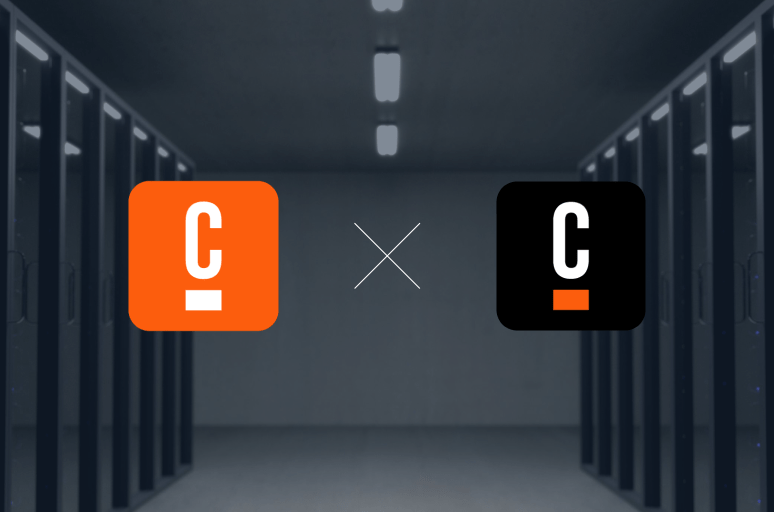
Process Automation
Camunda 7 vs Camunda 8 - key differences and considerations before migration
Explore the differences between Camunda 7 and Camunda 8. Understand key considerations for a smooth migration process.